22. Januar 2018 / Studierende
FIGGS-Seminar
Gaussian Process Latent Variable Model
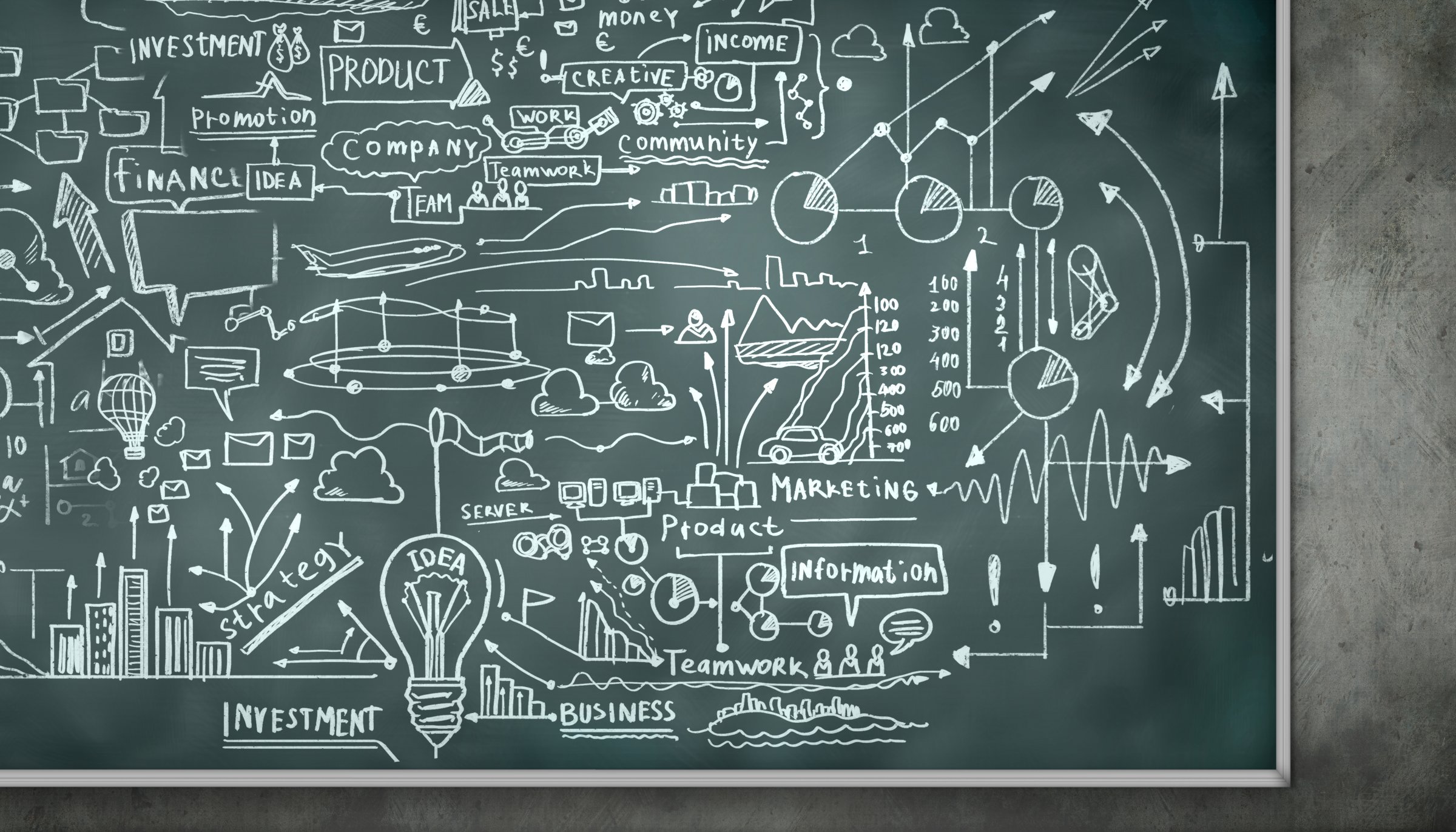
Sprecher: Raj Nirwan from the Systemic Risk Group
Abstract (das FIGGS-Seminar findet in englischer Sprache statt):
In many machine learning tasks, we have often to deal with very complexand high dimensional data. Many of the dimensions can be redundant and ourgoal can be to take care of this redundancy by learning a low dimensionalmanifold in the data-space. Many machine learning models have beenproposed to address this problem and are known as Latent Variable Models(LVMs). A well known algorithm for that purpose is the Principle ComponentAnalysis (PCA), which tries to find a linear manifold that captures thedata and minimize the reconstruction error. Keeping the number of latentdimensions fixed one can even further decrease the reconstruction error byallowing for non-linear manifolds.In this talk I am introducing a framework called Gaussian Process LatentVariable Model (GP-LVM), which is well applicable to non-lineardimensionality reduction. It is a combination of LVMs and Bayesiannon-parametric Gaussian Processes (GPs). After a short introduction to GPsand LVMs, I will give an intuitive interpretation to GP-LVMs and talkabout their application in real-world problems especially in finance forportfolio optimization.